How assuming placement and demand makes for better sales forecasting
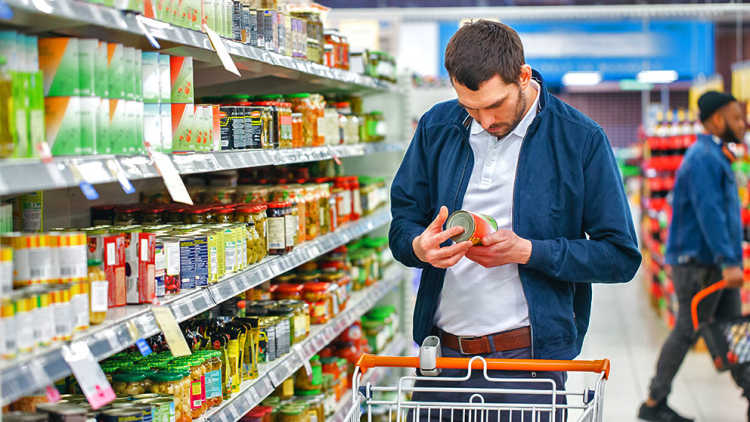
Written by Frank Luengo, chief operating officer of Sonora Foods Ltd.
There is a special feeling that comes from watching a customer choose to buy your brand. It’s a small confirmation that your product brings someone value.
But it’s only by repeating this activity multiple times per day, across hundreds or thousands of locations, distributed by networks of trucks and delivery routes, that you get the feeling that you have a scalable brand. Before you get there, you will need to have a vision for what this looks like, and you’ll need the numbers to back up that vision.
Long-term sales
In the previous introductory article on forecasting, I discussed some fundamental questions to ask when putting together a sales forecast, starting with demand and footprint. Demand assumptions get you scale, while footprint assumptions will allow you to estimate, from the bottom up, how many units of your product you will be able to sell over time.
First, consider the following top-down estimation question: how much chicken do Canadians eat in a year? If you make simple assumptions about population, consumption frequency and portion amounts, you can estimate that in a Canadian population of roughly 37.6 million, everyone consumes 800 grams of chicken a week, spread over lunch and dinner. That’s 41.6 kilograms per Canadian a year.
That was just a guess, and to show transparency, according to the Chicken Farmers of Canada, you’ll see that it is around 34.6 kilograms a year.
What’s your share of the market?
This top-down method gives you a sense of the size of pie that you’re going after with your new brand, such as “within five years, we want to capture 2% of the chicken market.” You will, of course, need to validate these assumptions with real market data, but this can get you far along with your analysis at a very low cost.
Bottom-up calculations
The next step is to estimate, bottom-up, the number of places your customer will purchase your product. Be as specific as you can be, with number of locations by major banners, independents and geographies. Segment all locations and filter out those that do not fit your brand or target consumer.
Once you have this number, estimate the number of SKUs and frequency of sales for each. In foodservice, you can look at menu research to see how many times your type of product is mentioned. In retail, you can buy sales data, which normally comes with past year growth rates. You can also undertake live consumer research, which is much more accessible today with the aid of technology and new research methods. Assign a timeline for when you think your products will be available at each location.
Apply a probability to your estimates
Now that you’ve built a model with locations, SKUs and turns, you should apply a probability to all your estimates in your forecast period. Early in the sales process, your probability should be low and should increase as specific stage gates are reached. By moving probabilities, SKUs and timelines around, you can now gauge the “sensitivity” of your sales forecast to your assumptions.
If you have a great product and plan, you will tend to over-estimate sales in the short term and under-estimate sales in the long term.
For example, before securing a meeting with a category manager, your probability might be 10% and after securing a meeting, 25%. If you get positive feedback from the category manager, move it up to 50%. Once you get verbal confirmation of a listing, move it up to 75%. The job is not done — you’re not at 100% — until the product goes through a full turn in the market and you get your second order.
This may seem daunting, but it is a critical exercise. Here’s some wisdom I’ve received over the years about estimation biases: If you have a great product and plan, you will tend to over-estimate sales in the short term and under-estimate sales in the long term. After all, we know that overnight successes often take years of hard work.
Bottom line
Building your forecast with demand and placement assumptions is a critical exercise. Estimate the size of the market you’re in, then calculate how many places customers can purchase your product and check sales data of similar products to see how much customers purchase. These elements combine to build a model for forecasting sales.
Frank Luengo is a Canadian food industry executive with a background and passion in corporate strategy and financing and has overseen the growth of Sonora Foods Ltd. as Chief Operating Officer since 2013. He holds a computer science degree from the University of Toronto and an MBA from the Rotman School of Business.
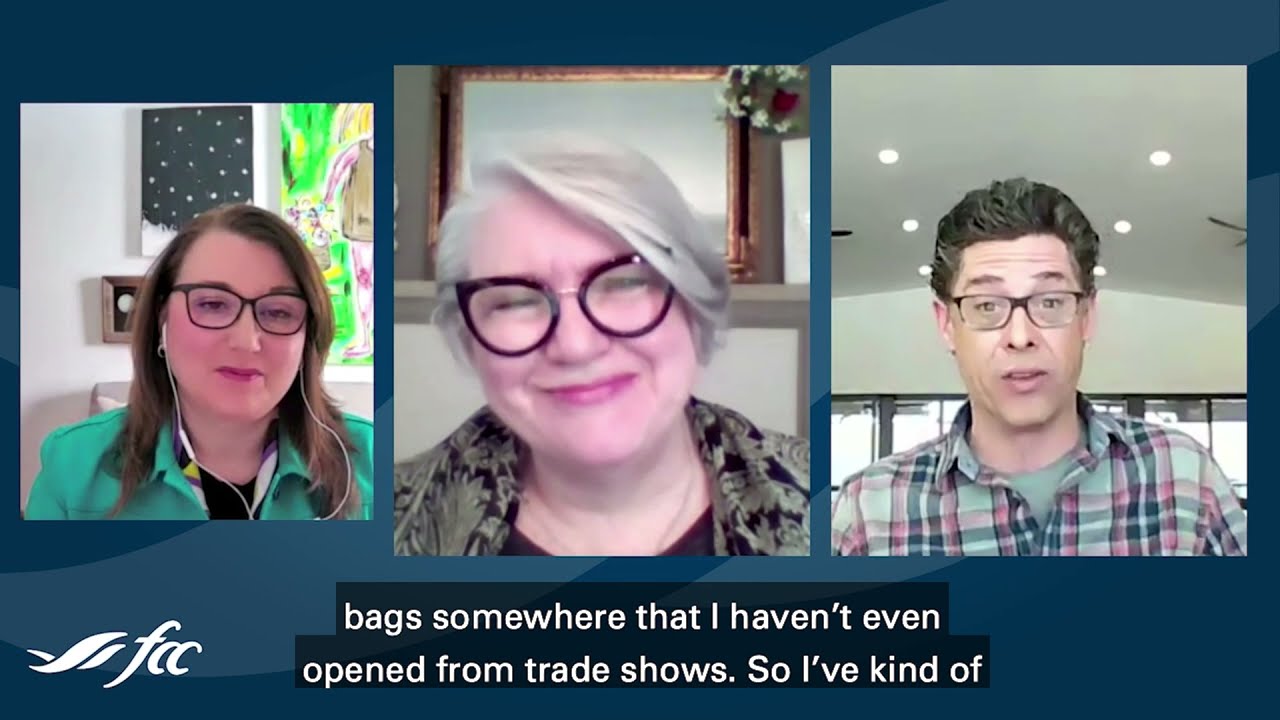
Learn to be ready for any question and have a follow-up plan when doing business at a trade show.